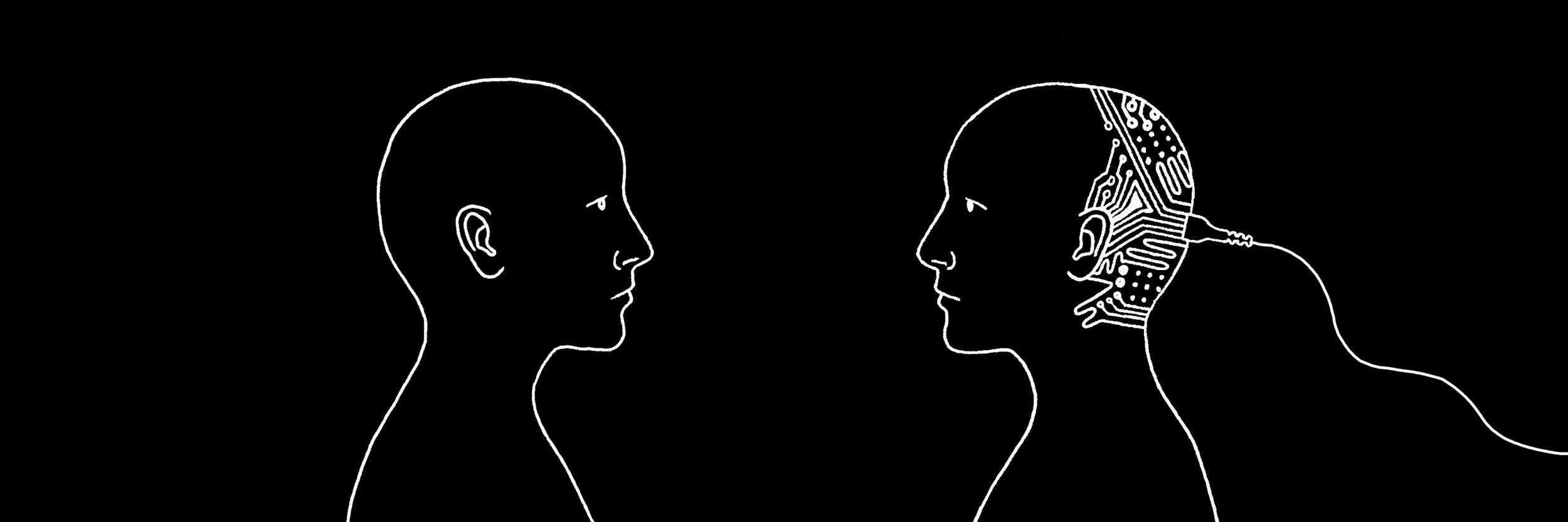
ABOUT
Machine deception refers to the capacity for machines to act as a medium through which human and other machine agents may be manipulated to believe, act upon or otherwise accept false information.
The development of machine deception has had a long, foundational impact on shaping research in the field of artificial intelligence. Thought experiments such as Alan Turing’s eponymous “Turing test” - where an automated system attempts to deceive a human judge into believing it is a human interlocutor, or Searle’s “Chinese room” - in which a human operator imbues the false impression of consciousness in a machine, are simultaneously exemplars of machine deception and some of the most famous and influential concepts in the field of AI.
As the field of machine learning advances, so too does machine deception seem poised to give rise to a host of practical opportunities and concerns. Recent demonstrations of techniques that synthesize hyper-realistic manipulations of audio and video - for instance - raise fundamental questions regarding the ability to preserve truth in the digital domain.
This NIPS workshop seeks to bring together the many technical researchers, policy experts, and social scientists working on different aspects of machine deception into conversation with one another. Our aim is to promote a greater awareness of the state of the research, and spark interdisciplinary collaborations as the field advances.
illustrations:
Machine Deceives Machine: Bot farms that automate posting on social media platforms to manipulate content ranking algorithms and spoof "relevance".
Human Deceives Machine: The planting of adversarial examples "in the wild" to exploit the fragility of autonomous systems and control their behavior.
Machine Deceives Human: The use of GANs to produce realistic manipulations of audio and video content that are indistinguishable from genuine recordings.

CALL FOR PAPERS
TOPICS OF INTEREST
Synthetic Media: ML systems increasingly learn representations that can be used to fabricate convincing simulations of authentic content. What are novel techniques and trends in generating synthetic media? Are there systematic tactics for detection?
Fooling the Machine: ML introduces a new set of vulnerabilities to both virtual and real-world systems. What is the next step for research in adversarial examples? What are other emerging classes of attack that are emerging?
Deceptive Agents: Bots have proven to be an increasingly popular means of shaping social behavior and spreading propaganda online. How might advancements in ML shape these uses going forwards, both in perpetrating these campaigns and combating them?
Policy and Ethics: How should law and regulation proceed, given the pace and trajectory of research into the use of ML for deceptive purposes? What norms should govern these applications in the research community?
Submitting a Paper
Papers submitted to the workshop should be up to four pages long excluding references and in NIPS 2017 format. They should be submitted on the EasyChair platform using this link. As the review process is not blind, authors can reveal their identity in their submissions. Accepted submissions will be presented as posters or talks. Demos accompanying the papers are encouraged.
Important Dates:
Submission deadline: 1 November 2017
Acceptance notification: 16 November 2017
Final paper submission: 5 December 2017
Workshop: 8 December 2017
Registration: The main NIPS 2017 conference is sold out. However authors of accepted papers will be able to register for the workshop sessions. We will notify authors prior to the cancellation deadline November 16.
Poster: Each accepted paper should bring a poster describing their work to the workshop. Poster dimensions are 36 x 48 inches (91cm x 122cm).
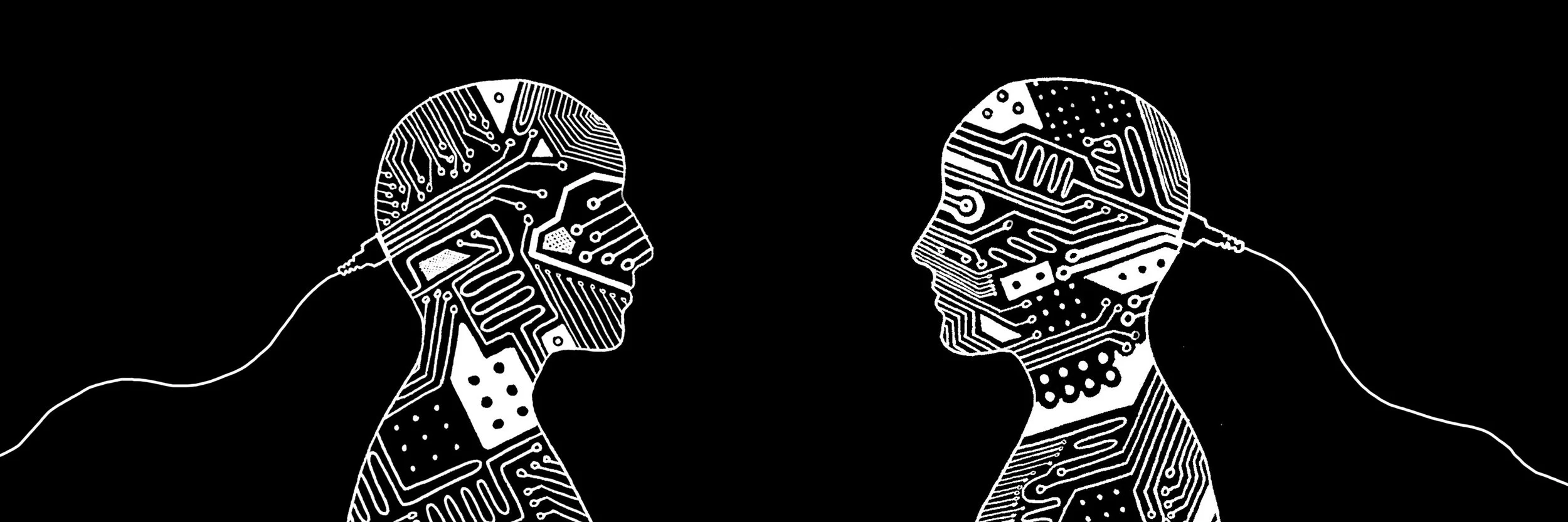
ORGANIZERS
Bryce Goodman - University of Oxford / DIUx
Tim Hwang - Ethics & Governance of AI Fund
Ian Goodfellow - Google Brain
Mikel Rodriguez - MITRE
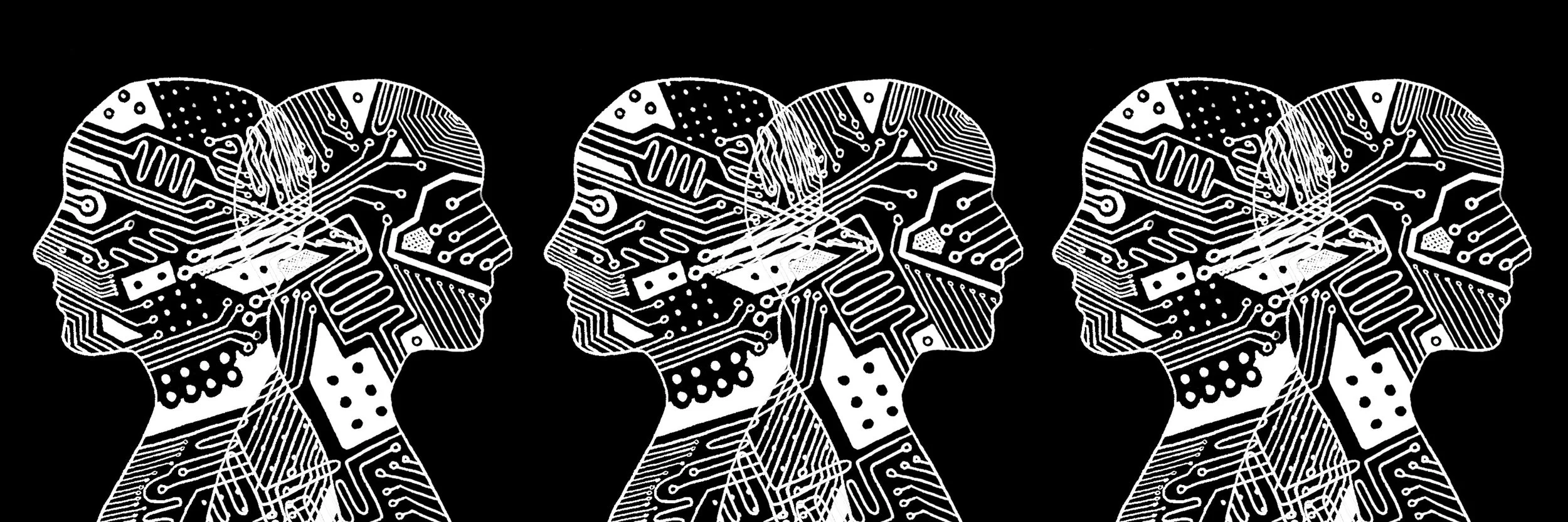
Contact
admin@machinedeception.com